Lorem ipsum dolor sit amet, consectetur adipiscing elit. Ut elit tellus, luctus nec ullamcorper mattis, pulvinar dapibus leo.
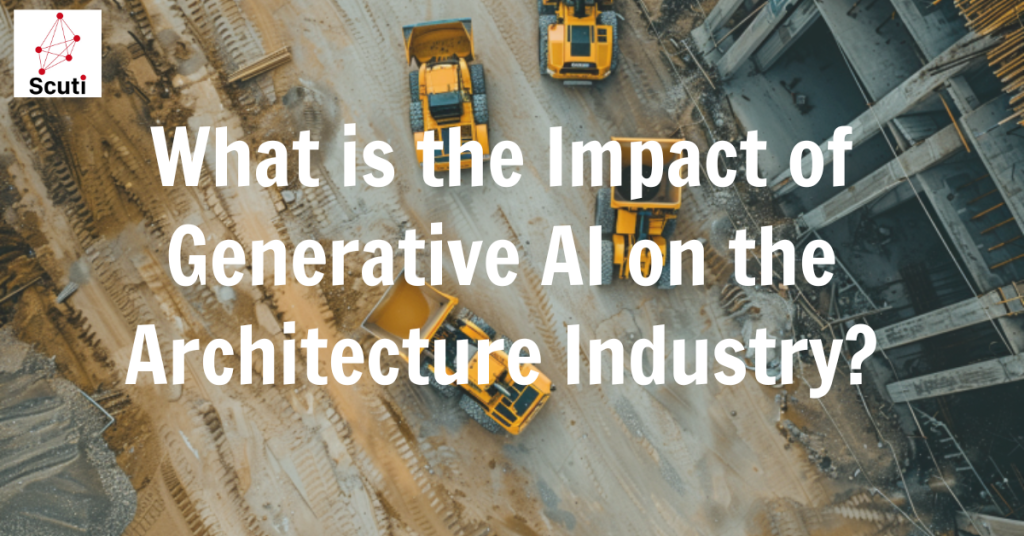
Hello, I am Kakeya, the representative of Scuti.
Our company specializes in Vietnamese offshore and lab-based development with a focus on generative AI. We also offer generative AI consulting services. Recently, we have been receiving a great number of requests for system development integrated with generative AI.
To all professionals in the architecture industry, are you aware of how generative AI can improve operational efficiency? Generative AI is being utilized across a broad range of areas, including design innovation, building predictive models, and optimizing operations.
This technology is not merely theoretical; it has shown tangible effects in actual projects. Particularly, through integration with BIM (Building Information Modeling), project management becomes more seamless, reducing errors and cutting costs.
Additionally, by combining it with technologies like IoT and deep learning, data-driven decision-making is achievable throughout the entire lifecycle of a building.
In this article, we will delve into how generative AI brings transformation to the architecture industry and enhances productivity.
If you would like to review the basics of what generative AI and ChatGPT are, please refer to the following articles:
- Introduction to Generative AI: A Comprehensive Guide to Text and Image Generation
- ChatGPT Beginner’s Guide: New Value Delivered by Generative AI
Basic Concepts and History of Generative AI
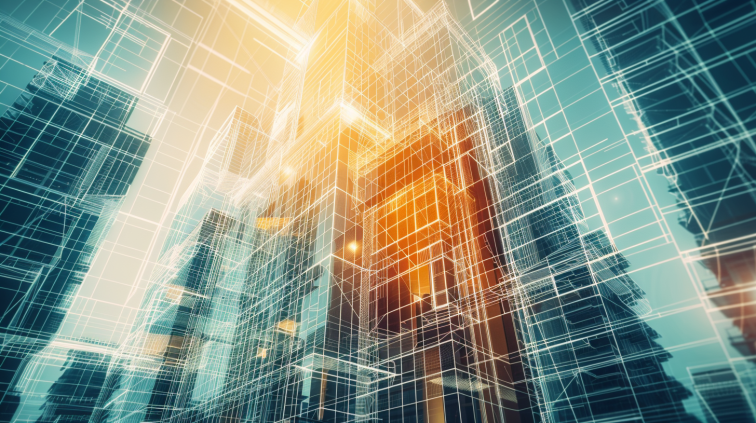
What is Generative AI? Its History and Characteristics
Generative AI refers to artificial intelligence technology capable of generating data. This technology enables the automatic creation of new designs, perspectives, images, and more. Generative AI has advanced dramatically with the evolution of neural networks.
Its key characteristics include:
- Automatic Generation Capability: It learns from data and can generate new content, significantly enhancing the efficiency of design and creative processes.
- Wide Range of Applications: Generative AI is utilized in various fields beyond the construction industry.
- High Flexibility: It can learn from multiple data sources and customize outputs to meet user needs.
Historically, the origins of generative AI date back to the 1980s. At that time, AI research mainly focused on rule-based systems, but machine learning methods began to emerge in the 1990s.
Subsequently, breakthroughs in deep learning led to remarkable progress in generative AI. Since the late 2010s, the advent of Generative Adversarial Networks (GANs) has enabled high-quality data generation, resulting in numerous practical applications.
The development of generative AI is expected to accelerate further in the future. In the construction industry, this technology holds significant potential for impact across all processes, from design to construction.
Development and Applications of Generative AI
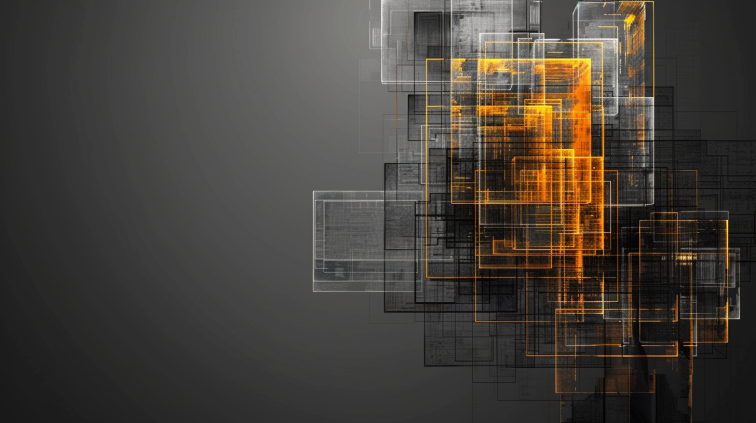
Examples of Generative AI Applications Across Various Industries
Generative AI has been developing and finding applications in a wide range of industries. Here, we will explain the trends in its development and specific examples of its application.
Development Trends: Generative AI has evolved primarily through advancements in deep learning and neural networks. In particular, the emergence of Generative Adversarial Networks (GANs) in 2014 greatly expanded the possibilities of generative AI. This technology has dramatically improved the accuracy and quality of data generation, making applications across industries possible.
Application Examples:
- Construction Industry: Generative AI is enhancing the efficiency of the design process through automated design and perspective generation. Its integration with BIM (Building Information Modeling) is expected to streamline project management, reduce errors, and cut costs.
- Automotive Industry: Generative AI is used in vehicle design and simulation. It contributes significantly to virtual prototype creation at the design stage and to the optimization of the manufacturing process.
- Medical Field: Generative AI plays a crucial role in medical imaging analysis and new drug development. For instance, it is used for the automatic detection of lesions and the generation of candidate compounds for new drugs.
- Entertainment Industry: In film and game production, generative AI is used to automatically create characters and scenes, reducing creators’ workload and allowing them to focus more on creative activities.
- Fashion Industry: Generative AI is used to create new designs and predict trends. It also proposes customized designs based on customer preferences.
Utilization of Generative AI in the Construction Field
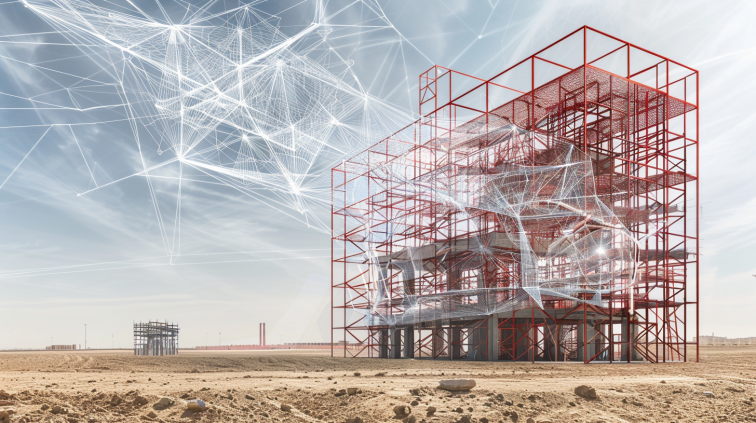
Innovation in the Design Process
Generative AI is significantly transforming the design process in the construction field, particularly through the automation and optimization of design.
- Design Automation: Generative AI is highly effective in the initial stages of architectural design. Tasks such as drawing creation and design proposals, traditionally done manually by designers, can now be automatically generated by AI. This allows designers to focus on more advanced creative tasks. For example, AI can study past design data and automatically generate new design proposals, enabling quick and diverse design suggestions.
- Design Optimization: Generative AI also serves as a tool for optimizing building performance. Specifically, it can simulate energy efficiency and structural strength of buildings, proposing optimal designs. This helps reduce environmental impact and cut costs. For instance, AI can suggest the ideal window placement considering sunlight and wind or propose structurally stable frame designs.
Additionally, integration with BIM (Building Information Modeling) allows for consistent management across the entire process from design to construction and maintenance, reducing errors and improving project efficiency. Utilizing generative AI in the early design stages also provides the flexibility to quickly respond to necessary modifications.
Integration of Generative AI and BIM
BIM plays a crucial role in managing and designing construction projects and is also an effective method to support the introduction of generative AI. This integration enables a streamlined process from design to construction and maintenance.
- Centralized Data Management: BIM centralizes all information related to a building as a digital model, including design drawings, structural information, material details, and construction schedules. Generative AI utilizes this vast data to propose new design options and optimal construction methods, improving design accuracy and facilitating smoother project progression.
- Efficiency in Design: The integration of BIM and generative AI greatly enhances the efficiency of the design process. By learning from BIM models, AI can generate optimal design proposals, alleviating the workload on designers. For instance, AI can analyze BIM models to automatically propose energy-efficient building designs or structurally stable designs, resulting in faster design processes and quality improvements.
- Optimization of Construction: Generative AI optimizes the construction process by utilizing BIM data. This includes optimizing construction schedules, efficient material allocation, and optimal workforce deployment. As a result, construction time and costs can be reduced. Furthermore, the integration of BIM and generative AI allows for real-time monitoring of issues during construction, enabling prompt responses.
- Improvement in Maintenance Management: BIM is also utilized in building maintenance. Based on BIM models, generative AI conducts data analysis across the entire lifecycle of a building, optimizing maintenance and proposing repair plans. This is expected to extend the lifespan of buildings and reduce operational costs.
Our Achievements in Generative AI Solutions
Our company has a track record of providing generative AI solutions for the construction industry, with the following examples:
- Data Structuring from Building Material Catalogs: Extracting information on building materials required for home construction manually from catalogs incurred substantial ongoing labor costs. To address this, we developed a system that reads catalogs with OCR, has generative AI interpret the extracted text, and then isolates building material data from unstructured catalog files for database structuring. This successfully reduced labor costs by over 70%.
- Document Search via AI Chat: Managing and sharing a vast amount of internal documents was challenging, making information retention and sharing highly dependent on individuals. To solve this, we built a secure RAG (Retrieval-Augmented Generation) system that enables smooth document sharing by searching and summarizing document information with generative AI. Note: Only text data is readable; diagrams and charts in design and technical documents are not included.
We have also created a guide titled “Immediate Applications of ChatGPT and Generative AI for the Construction Industry” for those in the construction field. It is available for free download, so please make use of it!
Download “Immediate Applications of ChatGPT and Generative AI for the Construction Industry” for Free
Optimization of Operations and Maintenance
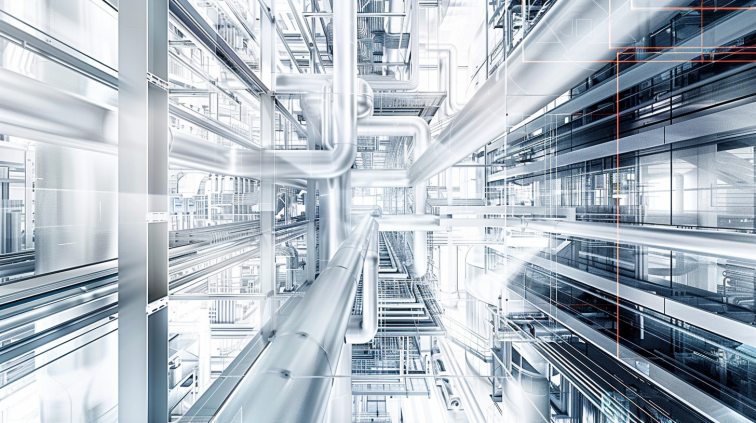
Benefits of Generative AI in Operations and Maintenance
Generative AI brings numerous benefits to the operation and maintenance of buildings. The main advantages are outlined below.
- Enabling Preventive Maintenance: Generative AI analyzes data collected from sensors and IoT devices in the building to detect early signs of equipment anomalies and failures. This enables scheduled maintenance and helps prevent unexpected breakdowns. For example, by analyzing data from air conditioning units and elevators, AI can identify anomalies early, allowing for timely maintenance.
- Cost Reduction: Generative AI also contributes to optimizing maintenance activities. By predicting maintenance needs and proposing optimal timing and methods, AI reduces unnecessary tasks and lowers costs. Specifically, it can extend equipment lifespan and avoid unnecessary purchases of replacement parts, thereby significantly reducing operational costs.
- Efficient Resource Management: Generative AI supports the optimization of maintenance schedules and resources. This enables efficient allocation of workers and optimal use of materials, accelerating the maintenance process. For instance, in the maintenance planning of large buildings, AI can suggest the ideal sequence of tasks and necessary resources, reducing work time and achieving efficient maintenance.
- Data-Driven Decision Making: Generative AI analyzes building operation data, providing insights that aid in optimizing operations. This enables data-driven decision-making and improves operational efficiency. For example, AI can analyze energy consumption data and propose energy efficiency improvements, reducing operational costs and environmental impact.
- Continuous Improvement: Through continuous analysis of operational data, generative AI constantly identifies and suggests improvements in operational processes. This leads to ongoing efficiency and quality enhancements, maximizing the building’s value. For instance, by periodically reviewing operational data, AI can propose new optimization measures, keeping the building’s performance in an optimal state.
Generative AI Implementation Process
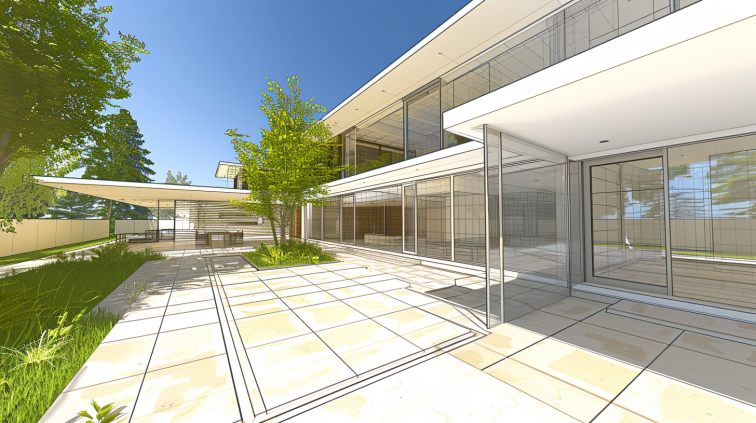
Specific Steps and Considerations
To implement generative AI in construction projects, several concrete steps and challenges need to be considered. The process is detailed below.
Specific Steps:
- Identifying Needs: Begin by clarifying the purpose and requirements for implementing generative AI, setting specific objectives such as design efficiency, cost reduction, or quality improvement.
- Data Collection and Preparation: Collect data for generative AI training, including past design, construction, and operational data. Clean and organize this data as needed.
- Model Selection and Development: Select and develop a generative AI model suited to the project’s needs. For instance, GANs are suitable for design automation, while RNNs are effective for forecasting.
- Training and Evaluation: Train the model using the collected data, then evaluate the trained model and improve it as necessary.
- Deployment and Operation: Deploy the model into the actual project after training and evaluation. Continuously monitor the model’s performance and adjust as needed.
Considerations:
- Data Quality and Quantity: High-quality, ample data is essential. If data is lacking or of low quality, AI performance may decline.
- Technical Knowledge and Skills: Specialized knowledge and skills are required to implement generative AI. If the organization lacks skilled personnel, external experts may need to be consulted.
- Cost and Time: Implementing generative AI requires initial investment. Additionally, model training and data preparation take time, so costs and time must be considered when planning implementation.
- Security and Privacy: It is essential to pay attention to security and privacy in data handling. When dealing with sensitive data, appropriate measures are crucial.
Technical Challenges and Solutions
There are several technical challenges in implementing generative AI. These challenges and their solutions are outlined below.
Technical Challenges:
- Data Quality and Quantity: The performance of generative AI heavily depends on the quality and quantity of training data. Insufficient or biased data can decrease AI accuracy.
- Securing Computing Resources: Generative AI training requires significant computational resources. Particularly with large datasets, powerful hardware is necessary.
- Lack of Expertise: Developing and operating generative AI requires advanced expertise. If skilled personnel are lacking, project progress may slow.
- Black Box Nature of Models: The internal workings of generative AI models are complex and challenging to understand, making results hard to interpret, which may reduce decision transparency.
Solutions:
- High-Quality Data Collection and Preprocessing: Improve data quality by gathering information from reliable sources and ensuring thorough data cleaning and preprocessing. Diverse datasets also help reduce bias.
- Utilization of Cloud Computing: To address computing resource challenges, use cloud computing, which provides flexible resource availability and improves cost efficiency.
- Collaboration with Experts: To address a lack of expertise, collaborate with external experts or consultants, enabling quick responses to technical issues.
- Implementation of Explainable AI Models: To ensure transparency, adopt explainable AI (XAI) technologies, which help make the decision-making processes of models more understandable.
Enhancing Safety and Quality
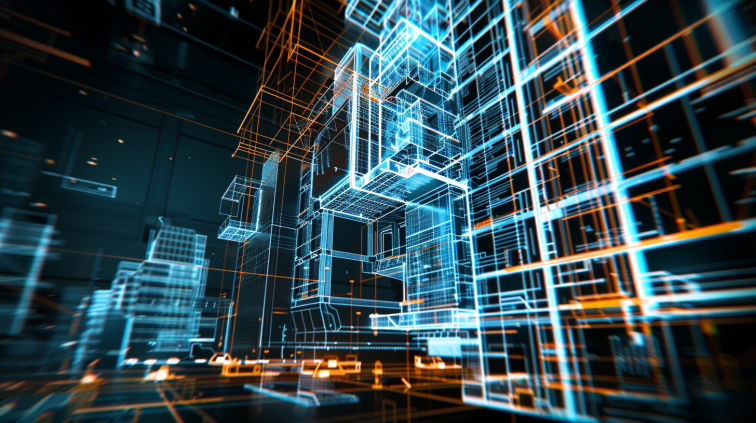
Methods for Improving Safety and Quality Management
Generative AI significantly contributes to improving safety and quality management in construction projects. Detailed methods are described below.
Enhancing Safety:
- Risk Prediction and Management: Generative AI analyzes site data and past accident data to predict potential risks, enabling preventive measures against accidents. For example, it can monitor workers’ movements and equipment usage in real time and propose appropriate actions to ensure safety.
- Monitoring Work Environment: AI analyzes data collected from site videos and sensors to detect hazardous situations early. This allows for timely responses before workers are exposed to danger. For instance, a system can be built to detect fall risks during high-altitude work or collision risks during heavy machinery operation in real time, issuing warnings as needed.
Improving Quality Management:
- Construction Quality Checks: Generative AI analyzes data from the construction process to detect quality issues early, enabling quick corrections for construction defects or design errors. For instance, an automated system can inspect aspects like wall straightness or concrete density, which are difficult to verify visually.
- Integration with BIM: Utilizing BIM data, AI checks if construction aligns with the design, minimizing discrepancies between planning and actual execution. For example, AI can compare design drawings with 3D scan data from the site to evaluate construction accuracy.
- Continuous Quality Improvement: Generative AI continuously analyzes construction and operational data, providing feedback for quality enhancement, leading to ongoing project quality improvement. For instance, by analyzing past project data, AI can identify recurring quality issues and implement solutions in future projects.
Research Trends and Future Prospects of Generative AI
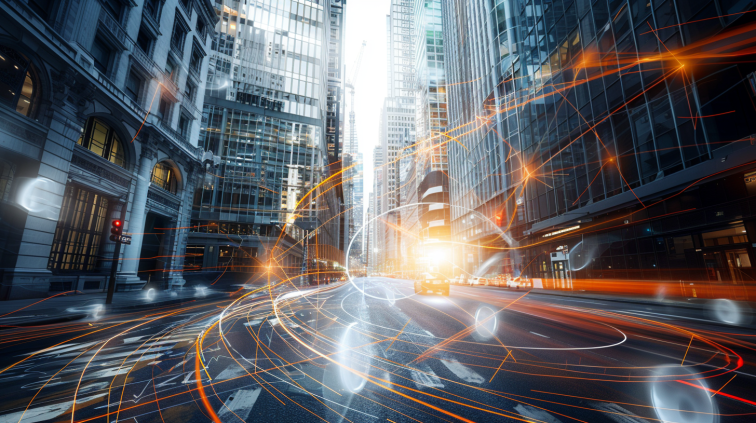
Research Gaps and Future Directions
While generative AI is evolving rapidly, there are still some unresolved challenges in its research. These challenges and the future directions for research are detailed below.
Research Gaps:
- Data Bias: The performance of generative AI heavily depends on the quality of its training data. Biases within datasets can influence the generated outcomes. In architectural design, for instance, data biased toward specific styles or cultures may affect the results.
- Lack of Interpretability: Generative AI models are highly complex, resulting in a “black box” issue that makes understanding their mechanisms difficult. This complexity makes it challenging to explain the rationale behind generated designs or suggestions.
- Limitations in Computational Resources: Generative AI models require extensive computational resources for training. This raises research and development costs, making it challenging for resource-limited organizations and research institutions to adopt these models.
Future Directions:
- Enhancing Data Diversity and Reducing Bias: In generative AI research, methods to ensure dataset diversity and reduce bias are essential. Specifically, building datasets that encompass various cultures and styles, along with developing algorithms for bias detection and correction, is needed.
- Development of Explainable AI Models: To improve transparency in generative AI, research on interpretable models and explainable AI (XAI) is crucial. This would clarify the rationale behind generated results, enhancing user trust.
- Development of Efficient Computational Methods: To overcome computational resource constraints, efficient algorithms and the use of distributed computing are necessary. This would enable high-performance generative AI even in resource-constrained environments.
- Consideration of Ethical and Social Impacts: As generative AI becomes more widespread, research on its ethical and social impacts is also important. Evaluating the societal impact of AI-generated designs and proposals and establishing ethical guidelines will be essential.