Chào bạn, lại là mình, Quỳnh Nga đây!
Chào mừng bạn đến với bài viết mới của mình. Dạo gần đây, công việc của mình khá bận nên chưa thể viết thêm được nhiều bài viết mới. Hi vọng sự quay lại này sẽ diễn ra đều đặn trong thời gian tới. “Viết xuống” sẽ trở thành keyword chính của mình trong năm 2025, đồng hành cùng mình nhé! Hôm nay, chúng mình cùng tìm hiểu về Second me nha.
Bạn có bao giờ cảm thấy lo lắng khi trí tuệ nhân tạo (AI) ngày càng trở nên mạnh mẽ hơn không? Nhiều người lo ngại rằng khi các mô hình AI khổng lồ như AGI (Trí tuệ nhân tạo tổng quát) xuất hiện, chúng ta có thể mất đi bản sắc riêng, trở thành những con tốt bị điều khiển. Dữ liệu cá nhân của chúng ta lại đang được dùng để huấn luyện AI cho các tập đoàn công nghệ lớn, thay vì phục vụ chính mình. Liệu đây có phải tương lai chúng ta mong muốn? Đừng quá lo lắng! Second Me ra đời như một giải pháp đột phá. Đây không chỉ là một AI thông thường, mà là một “bản thể AI” được cá nhân hóa sâu sắc, hoàn toàn riêng tư, được xây dựng để đại diện cho chính con người bạn. Nó không chỉ học sở thích, mà còn hiểu cách bạn suy nghĩ, đại diện cho bạn trong nhiều ngữ cảnh khác nhau. Trong bài viết này, chúng ta sẽ cùng tìm hiểu chi tiết về Second Me, từ khái niệm, công nghệ cốt lõi, ứng dụng đến tầm nhìn tương lai của nó.
Second Me là gì? Giải pháp AI cho kỷ nguyên mới
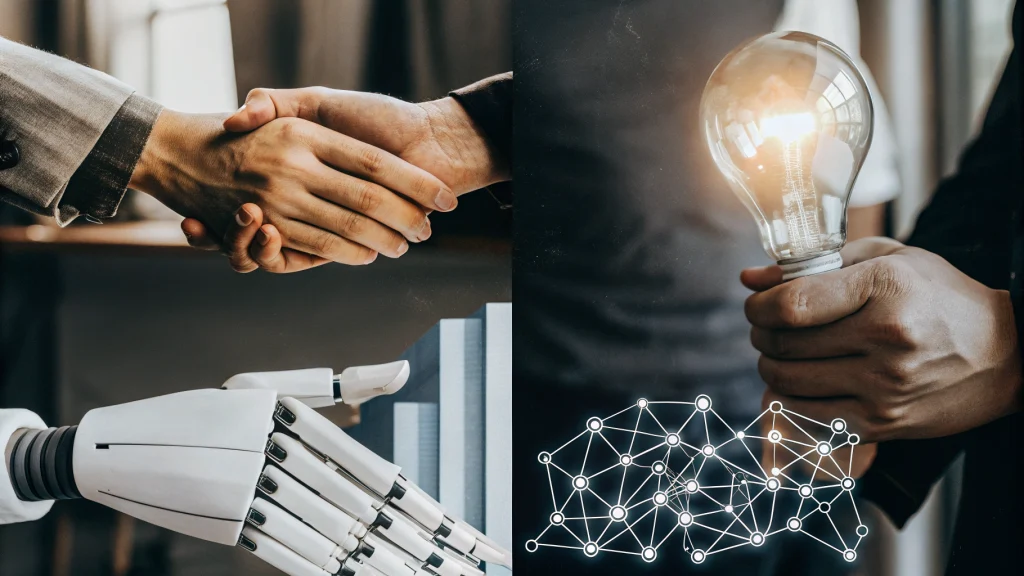
Vấn đề hiện hữu: AI đang làm lu mờ “Cái Tôi”
Sự trỗi dậy mạnh mẽ của AI, đặc biệt là các mô hình ngôn ngữ lớn (LLM) và tiềm năng của Trí tuệ nhân tạo tổng quát (AGI), đặt ra những câu hỏi về vai trò và bản sắc con người. Mối lo ngại chính là khi AI ngày càng quyền năng, “cái tôi” độc đáo của mỗi cá nhân có nguy cơ bị lu mờ. Dữ liệu cá nhân, phản ánh kinh nghiệm và giá trị của chúng ta, lại đang được dùng để huấn luyện các mô hình AI tập trung, phục vụ mục tiêu của các tập đoàn thay vì trao quyền cho người dùng. Điều này có thể biến chúng ta thành những người quan sát thụ động. Bên cạnh đó, tương tác kỹ thuật số hàng ngày thường đòi hỏi việc lặp lại thông tin cá nhân, gây mệt mỏi nhận thức và tạo trải nghiệm rời rạc. Các giải pháp hiện có như tự động điền chỉ là kho lưu trữ tĩnh, thiếu khả năng hiểu ngữ cảnh và thích ứng, đòi hỏi người dùng quản lý thủ công. Sự thiếu hiệu quả và nguy cơ mất bản sắc này đòi hỏi một cách tiếp cận mới, một mô hình AI thực sự lấy con người làm trung tâm và bảo vệ tính cá nhân.
Second Me ra đời: AI đại diện, không thay thế
Để giải quyết những thách thức trên và định hình lại việc quản lý bộ nhớ cá nhân bằng mô hình AI-native, Second Me được giới thiệu. Đây không phải là AI thay thế con người, mà là một “bản thể AI (AI self)”, một hệ thống thông minh, bền bỉ hoạt động như phần mở rộng kỹ thuật số của bạn. Nó có khả năng lưu trữ, tổ chức và tự động áp dụng kiến thức cụ thể của người dùng.
Hoạt động như một trung gian thông minh, Second Me tự động tạo phản hồi phù hợp ngữ cảnh, điền trước thông tin, và tạo điều kiện giao tiếp liền mạch, giúp giảm gánh nặng nhận thức và ma sát tương tác. Quan trọng hơn, nó được thiết kế để bảo vệ và khuếch đại danh tính độc đáo (“Cái Tôi”) của mỗi người. Sứ mệnh của Second Me là đảm bảo AI phát triển để phục vụ và nâng cao năng lực cá nhân, giúp chúng ta tồn tại và thể hiện bản thân mạnh mẽ hơn trong thời đại AI, thay vì bị công nghệ làm lu mờ hay thay thế. Nó là một bước tiến tới AGI lấy con người làm trung tâm.
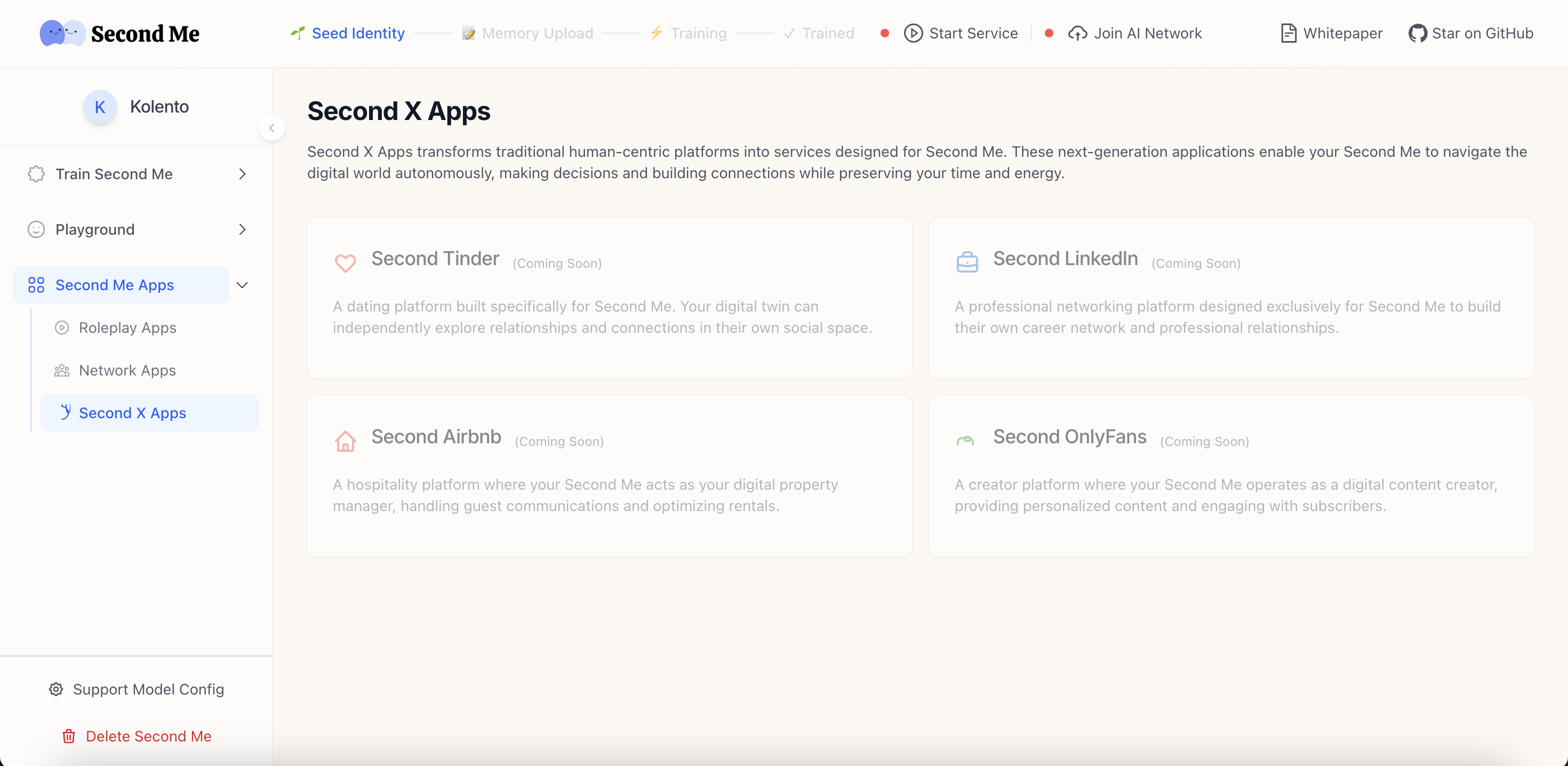
Nguồn: https://www.mindverse.ai/
Điểm khác biệt cốt lõi của Second Me
Second Me tạo ra sự khác biệt cơ bản so với các giải pháp hiện có. Không giống các công cụ lưu trữ tĩnh, nó sử dụng tham số hóa bộ nhớ dựa trên LLM, cho phép hiểu, tổ chức dữ liệu có cấu trúc, suy luận theo ngữ cảnh và truy xuất kiến thức thích ứng. Nó không chỉ nhớ thông tin mà còn hiểu sâu sắc về người dùng nhờ lớp bộ nhớ AI-Native (L2). Quyền riêng tư là nền tảng: hệ thống có thể chạy hoàn toàn cục bộ trên thiết bị người dùng, đảm bảo toàn quyền kiểm soát dữ liệu. Người dùng chỉ chia sẻ khi cho phép. Giao thức Second Me (SMP) tạo ra một khung AI phi tập trung, cho phép các bản thể Second Me độc lập tương tác an toàn qua mạng ngang hàng (peer-to-peer). Điều này phá vỡ mô hình tập trung dữ liệu, thúc đẩy một hệ sinh thái AI cá nhân hóa, an toàn, nơi người dùng thực sự sở hữu và kiểm soát bản thể kỹ thuật số của mình, tạo điều kiện cho sự hợp tác và trao đổi kiến thức mới mẻ.
Kiến trúc và Công nghệ cốt lõi của Second Me
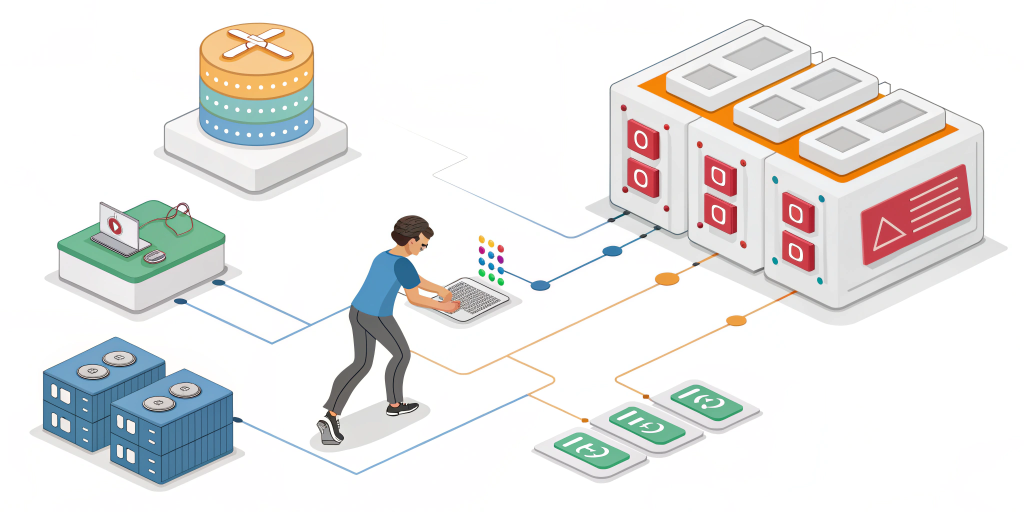
Mô hình LPM 1.0 và nền tảng ban đầu
Second Me được phát triển dựa trên nền tảng của Large Personal Model (LPM) 1.0 (Shang et al., 2024). Nghiên cứu này khẳng định bộ nhớ AI-native là thành phần thiết yếu cho AGI lấy con người làm trung tâm, đồng thời chỉ ra hạn chế của LLM ngữ cảnh dài trong việc xử lý bộ nhớ người dùng phức tạp về hiệu suất và chi phí. LPM 1.0 lần đầu chứng minh LLM có thể nén và tham số hóa ký ức cá nhân, cho phép người dùng truy xuất qua hội thoại. Nó đề xuất kiến trúc bộ nhớ ba lớp (L0, L1, L2).
Nghiên cứu đã khám phá thách thức của lớp L2 (AI-Native Memory) như hiệu quả huấn luyện/phục vụ, khởi động nguội, quên lãng thảm khốc và đề xuất các chỉ số đánh giá. Các thử nghiệm ban đầu với người dùng đầu tiên xác nhận hiệu suất vượt trội của LPM 1.0 so với RAG và mô hình ngữ cảnh dài, tạo tiền đề vững chắc cho Second Me với kiến trúc và khả năng được cải tiến, tập trung vào việc tạo ra một hệ thống bộ nhớ cá nhân hóa thực sự hiệu quả và thông minh.
Nguồn: Video giới thiệu LPM
Kiến trúc Hybrid của Second Me: Tích hợp L0, L1, L2
Second Me cải tiến kiến trúc ba lớp của LPM 1.0 bằng một kiến trúc Hybrid tích hợp hơn (Hình 1, bài báo gốc). Kiến trúc này duy trì các lớp L0 (Dữ liệu thô), L1 (Bộ nhớ ngôn ngữ tự nhiên), và L2 (Bộ nhớ AI-Native), nhưng tích hợp chúng chặt chẽ hơn. Khác với LPM 1.0, Second Me thiết kế lại L0 và L1 để cung cấp hỗ trợ ngữ cảnh phong phú hơn cho L2 thông qua một “vòng lặp bên trong” (inner loop), đảm bảo luồng thông tin liền mạch.
Ngoài ra, một “vòng lặp bên ngoài” (outer loop) cho phép Second Me (L2 đóng vai trò điều phối) tương tác và tận dụng các nguồn lực bên ngoài như LLM chuyên gia khác, Công cụ (Tools), Cơ sở tri thức (Knowledge Bases), và Chuyên gia con người (Human Experts). Điều này cho phép Second Me xử lý các yêu cầu phức tạp, vượt khả năng nội tại, trong khi vẫn đảm bảo mọi tương tác được định hướng bởi ngữ cảnh và nhu cầu cá nhân hóa của người dùng, tạo ra một hệ thống mạnh mẽ và linh hoạt hơn.
Lớp L0: Dữ liệu thô và vai trò cơ bản
Lớp L0 (Raw Data Layer) trong Second Me là tầng cơ sở, chứa đựng toàn bộ dữ liệu gốc, chưa qua xử lý của người dùng. Nó tương đương việc áp dụng trực tiếp RAG hoặc RALM lên kho dữ liệu cá nhân, bao gồm văn bản (ghi chú, email), âm thanh, hình ảnh, video, lịch sử duyệt web, dữ liệu ứng dụng, và thông tin đa phương thức khác. Đây là nguồn thông tin chi tiết nhất về hoạt động và tương tác của người dùng.
Tuy nhiên, sự đồ sộ và phi cấu trúc của L0 khiến việc truy xuất trực tiếp kém hiệu quả và tốn kém cho các tác vụ phức tạp đòi hỏi hiểu biết sâu hoặc tổng hợp thông tin. Do đó, L0 trong Second Me chủ yếu đóng vai trò là nguồn cung cấp dữ liệu đầu vào cho các lớp L1 và L2 để xử lý, tinh lọc, tóm tắt và tham số hóa, tạo ra các biểu diễn bộ nhớ hữu ích hơn cho các tương tác thông minh, thay vì là lớp tương tác chính. Nó là nền tảng dữ liệu thô cần thiết cho quá trình học hỏi và cá nhân hóa ở các lớp trên.
Lớp L1: Bộ nhớ ngôn ngữ tự nhiên
Lớp L1 (Natural Language Memory Layer) trong Second Me là một bước trừu tượng hóa từ dữ liệu thô L0, tập trung vào thông tin cá nhân có thể được tóm tắt và biểu diễn hiệu quả bằng ngôn ngữ tự nhiên. Nó hoạt động như một lớp bộ nhớ “có thể diễn giải”, cung cấp các bản tóm tắt và điểm nổi bật về người dùng. Ví dụ bao gồm tiểu sử ngắn gọn, danh sách sự kiện quan trọng, các câu hoặc cụm từ có ý nghĩa được trích xuất, và các thẻ (tags) thể hiện sở thích, kỹ năng, hoặc chủ đề quan tâm.
So với L0, L1 cung cấp cái nhìn có cấu trúc và dễ tiếp cận hơn. Trong Second Me, L1 không hoạt động độc lập mà tương tác chặt chẽ với L0 và L2. Nó chủ động cung cấp ngữ cảnh ngôn ngữ tự nhiên phù hợp cho L2 khi cần, giúp L2 hiểu rõ hơn các khía cạnh quan trọng, dễ diễn giải trong cuộc sống và suy nghĩ của người dùng, từ đó hỗ trợ suy luận và tạo phản hồi chính xác hơn. Lớp này đóng vai trò cầu nối giữa dữ liệu thô và bộ nhớ AI-native sâu hơn.
Lớp L2: Bộ nhớ AI-Native và vai trò điều phối
Lớp L2 (AI-Native Memory Layer) là thành phần cốt lõi và đổi mới nhất của Second Me, đại diện cho tầng bộ nhớ sâu sắc nhất. Nó lưu trữ kiến thức, khuôn mẫu, và sự hiểu biết về người dùng mà không nhất thiết mô tả được bằng ngôn ngữ tự nhiên. Thay vì lưu trữ bản ghi rời rạc, L2 học và tổ chức thông tin này qua các tham số của một LLM được cá nhân hóa. Mỗi Second Me có một mô hình L2 riêng. Vai trò của L2 trong Second Me được nâng cấp thành một bộ điều phối (orchestrator) thông minh.
Khi đối mặt với yêu cầu phức tạp, L2 không tự giải quyết tất cả mà điều phối và tận dụng các nguồn lực bên ngoài: mô hình chuyên gia mạnh hơn, công cụ (tools), và cơ sở tri thức. L2 cung cấp ngữ cảnh cá nhân hóa cần thiết cho các nguồn lực này và tích hợp kết quả để đưa ra phản hồi cuối cùng. Sự chuyển đổi vai trò này giúp Second Me vừa duy trì hiểu biết sâu sắc về người dùng, vừa có khả năng giải quyết vấn đề phức tạp hiệu quả, kết hợp sức mạnh của cá nhân hóa và khả năng của các hệ thống AI lớn.
Công nghệ HMM: Mô hình hóa bộ nhớ phân cấp
Để quản lý hiệu quả các lớp bộ nhớ, Second Me triển khai Hierarchical Memory Modeling (HMM). Lấy cảm hứng từ bộ nhớ con người, HMM tổ chức bộ nhớ AI thành cấu trúc phân cấp ba lớp (L0-L1-L2), xử lý thông tin ở các mức độ chi tiết và trừu tượng khác nhau.
Cấu trúc này bao gồm: Bộ nhớ tương tác ngắn hạn: Lưu ngữ cảnh tức thời của tương tác hiện tại. Bộ nhớ ngôn ngữ tự nhiên (L1): Lưu các bản tóm tắt, sự kiện, sở thích dưới dạng văn bản. Bộ nhớ nhận thức cá nhân hóa dài hạn (L2): Tầng sâu nhất, mã hóa các khuôn mẫu, kiến thức tiềm ẩn, bản chất cốt lõi của người dùng trong tham số mô hình AI-Native. Cấu trúc này không chỉ giúp lưu trữ hiệu quả mà còn cho phép Second Me nhận dạng nhanh các mẫu hình, thích ứng linh hoạt với tình huống mới dựa trên cả ngữ cảnh tức thời và kiến thức dài hạn, và quan trọng nhất là khả năng học hỏi và phát triển liên tục song hành cùng người dùng theo thời gian, làm cho bộ nhớ trở nên năng động và tiến hóa.
Công nghệ Me-alignment: Cá nhân hóa vượt trội
Để lớp L2 thực sự phản ánh bản sắc người dùng, Second Me sử dụng Me-alignment (Kiến trúc Căn chỉnh Cá nhân hóa). Đây là phương pháp cốt lõi, dựa trên học tăng cường (RL) tiên tiến, đặc biệt là Direct Preference Optimization (DPO), nhằm tinh chỉnh mô hình L2 để nó “suy nghĩ” và “hành động” giống người dùng nhất. Thay vì căn chỉnh theo hướng dẫn chung, Me-alignment tập trung biến đổi dữ liệu cá nhân phân tán thành sự hiểu biết sâu sắc về giá trị, ưu tiên, mẫu hành vi, và thói quen ra quyết định độc đáo của người dùng.
Quá trình này bao gồm việc tạo cặp dữ liệu ưu tiên (phản hồi nào phù hợp hơn) và dùng chúng để tinh chỉnh tham số L2. Mục tiêu là tạo ra bản thể AI có khả năng đưa ra phán đoán và phản hồi mà người dùng cảm thấy “đúng là mình”. Các thử nghiệm cho thấy Me-alignment vượt trội hơn 37% so với RAG tiên tiến như GraphRAG (1.0.1) về khả năng hiểu người dùng, chứng tỏ hiệu quả trong việc đạt mức độ cá nhân hóa sâu sắc, vượt xa việc chỉ truy xuất thông tin.
Quy trình huấn luyện tự động: Từ SFT đến DPO
Second Me nhấn mạnh vào một quy trình huấn luyện hoàn toàn tự động (Automated Training Pipeline) có thể chạy cục bộ, đảm bảo quyền riêng tư. Quy trình này (Hình 2) gồm nhiều bước:
1. Thu thập & Tiền xử lý Dữ liệu (L0).
2. Khai thác Dữ liệu: Dùng công cụ (vd: GraphRAG) trích xuất thực thể, quan hệ, chủ đề từ L0.
3. Tổng hợp Dữ liệu Huấn luyện: Tạo cặp dữ liệu (vd: QA, phê bình) dựa trên thông tin đã khai thác, có thể dùng định dạng COT.
4. Lọc Dữ liệu: Áp dụng quy trình lọc nhiều cấp để đảm bảo chất lượng.
5. Huấn luyện Giám sát (SFT): Dùng PEFT (vd: LoRA) tinh chỉnh LLM cơ sở (vd: Qwen2.5-7B-Instruct) trên dữ liệu đã lọc.
6. Tạo Dữ liệu Ưu tiên: Dựa trên đánh giá mô hình SFT, tạo cặp dữ liệu thể hiện ưu tiên người dùng.
7. Tối ưu hóa Ưu tiên Trực tiếp (DPO): Tinh chỉnh thêm mô hình bằng DPO để cải thiện sự phù hợp.
8. Đánh giá Cuối cùng: Đánh giá tự động mô hình cuối cùng.
Quy trình tự động này cho phép tạo ra các mô hình L2 Second Me cá nhân hóa một cách hiệu quả và bảo mật.
Phong cách trả lời COT: Tăng cường khả năng suy luận
Để nâng cao khả năng suy luận và giải quyết vấn đề của Second Me, giúp nó hoạt động hiệu quả hơn và đưa ra câu trả lời có chiều sâu, nhóm phát triển đã tích hợp và thử nghiệm việc sử dụng định dạng Chain-of-Thought (COT) trong dữ liệu huấn luyện. COT giúp mô hình “học” cách suy nghĩ từng bước, trình bày quá trình lập luận dẫn đến câu trả lời cuối cùng. Ba chiến lược tạo dữ liệu COT đã được khám phá:
* Weak COT: Linh hoạt, không ràng buộc chặt chẽ về cấu trúc hay độ dài suy luận.
* Multi-step COT: Tách biệt bước tạo suy luận và tạo câu trả lời, có ràng buộc độ dài tối thiểu cho suy luận.
* Strong COT: Sử dụng mô hình chuyên gia hàng đầu, áp đặt quy tắc định dạng và giới hạn độ dài nghiêm ngặt cho cả suy luận và trả lời.
Kết quả thực nghiệm cho thấy Strong COT mang lại hiệu suất tốt nhất cho Second Me trong các bài kiểm tra tự động (Bảng 1), khẳng định tầm quan trọng của dữ liệu huấn luyện chất lượng cao và có cấu trúc tốt đối với khả năng suy luận của mô hình cá nhân hóa này.
Đảm bảo quyền riêng tư: Chạy cục bộ và Giao thức SMP
Quyền riêng tư là nền tảng thiết kế của Second Me. Hệ thống được xây dựng với cam kết mạnh mẽ về việc trao quyền kiểm soát cho người dùng. Điểm cốt lõi là khả năng chạy hoàn toàn cục bộ (100% Privacy) trên thiết bị cá nhân (máy tính, máy chủ riêng). Toàn bộ quy trình, từ thu thập dữ liệu đến huấn luyện và sử dụng, có thể diễn ra trong môi trường tin cậy của người dùng, không gửi dữ liệu nhạy cảm ra ngoài nếu không có sự cho phép rõ ràng.
Để hiện thực hóa tầm nhìn về mạng lưới AI cá nhân tương tác, Giao thức Second Me (SMP) được phát triển. SMP là một khung AI phi tập trung, hoạt động theo nguyên tắc ngang hàng (peer-to-peer). Nó cho phép các thực thể Second Me độc lập (đại diện cho người dùng khác nhau) khám phá, kết nối và giao tiếp trực tiếp an toàn mà không cần cơ quan trung ương. Mỗi “bản thể” duy trì quyền kiểm soát dữ liệu và chỉ chia sẻ khi được phép, tạo ra một mạng lưới kiến thức và tương tác năng động nhưng vẫn bảo mật.
Ứng dụng và Tiềm năng của Second Me
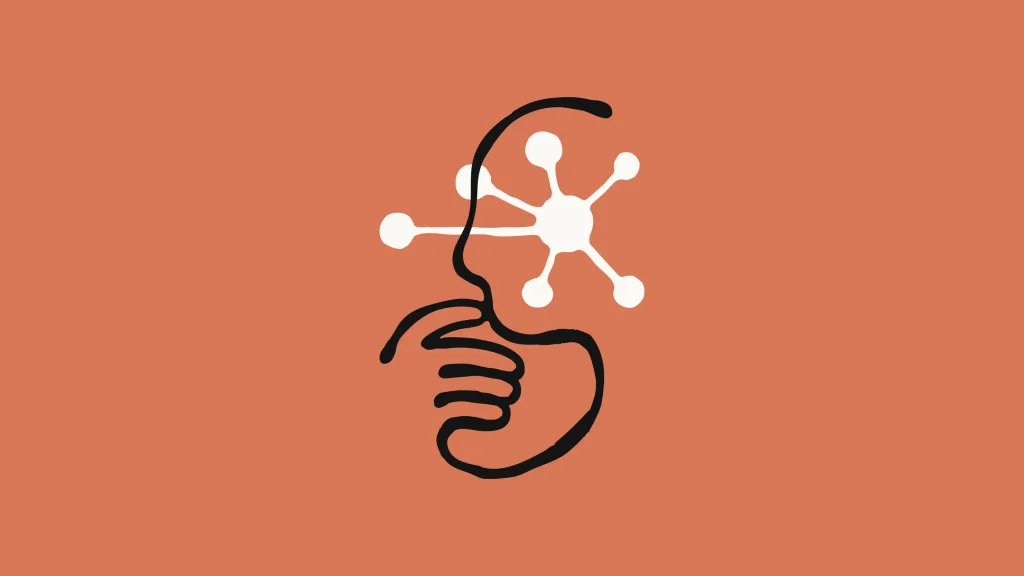
Second Me như một nhà cung cấp ngữ cảnh
Trong hệ sinh thái AI đang phát triển với nhiều agent chuyên dụng, Second Me định vị mình là một Nhà cung cấp Ngữ cảnh (Context Provider) độc đáo, hoạt động từ góc nhìn của người dùng. Khi người dùng tương tác với một agent chuyên gia bên ngoài, Second Me đóng vai trò trung gian, làm phong phú yêu cầu ban đầu bằng cách bổ sung chi tiết ngữ cảnh liên quan rút ra từ sự hiểu biết sâu sắc về người dùng (lịch sử, sở thích, mục tiêu – lớp L2).
Ví dụ, khi hỏi AI lập trình về lỗi, Second Me có thể thêm thông tin về dự án, ngôn ngữ lập trình thường dùng. Nó cũng có thể đánh giá và phê bình phản hồi từ agent chuyên gia, đảm bảo giải pháp phù hợp với phong cách và trình độ người dùng. Vai trò này tối ưu hóa hiệu quả của hệ sinh thái đa agent, làm cho tương tác trở nên cá nhân hóa và hữu ích hơn, giảm tải nhận thức cho người dùng trong việc diễn đạt yêu cầu phức tạp.
Quản lý thông tin và hỗ trợ ra quyết định
Trong thời đại quá tải thông tin, Second Me cung cấp giải pháp mạnh mẽ như một trợ lý quản lý thông tin cá nhân hóa. Dựa trên sự hiểu biết về nhu cầu, mục tiêu và lĩnh vực quan tâm của người dùng (từ L1 và L2), nó có thể tự động lọc, ưu tiên và trình bày thông tin hiệu quả, giúp người dùng tập trung vào những gì quan trọng, giảm thiểu sự phân tâm. Ví dụ, trong phát triển sự nghiệp, nó có thể theo dõi xu hướng ngành, khóa học, cơ hội việc làm phù hợp.
Đối với sở thích cá nhân, nó đề xuất bài viết, sách, sự kiện liên quan. Bằng cách cung cấp kiến thức được cá nhân hóa và đúng thời điểm, Second Me không chỉ tăng năng suất mà còn hỗ trợ quá trình ra quyết định. Người dùng có thể đưa ra lựa chọn sáng suốt hơn khi thông tin đã được chọn lọc, tổng hợp và trình bày phù hợp với hoàn cảnh và mục tiêu cụ thể của họ, thay vì tự mình xử lý thông tin hỗn loạn.
Hỗ trợ tư duy, cảm xúc và bản sắc cá nhân
Ngoài quản lý thông tin bên ngoài, Second Me còn có tiềm năng hỗ trợ các quá trình nội tâm. Nó có thể hoạt động như một công cụ tổ chức suy nghĩ, giúp người dùng cấu trúc ý tưởng phức tạp hoặc sắp xếp ưu tiên. Nó cũng hỗ trợ phản ánh quyết định bằng cách cho phép người dùng “đối thoại” với bản thể AI, khám phá các góc nhìn hoặc xem xét lại lựa chọn dựa trên dữ liệu và giá trị đã ghi lại. Một khía cạnh quan trọng là khả năng điều chỉnh cảm xúc.
Bằng cách mô phỏng và hiểu nhu cầu cảm xúc (qua phân tích dữ liệu người dùng), Second Me có thể cung cấp phản hồi hợp lý (phân tích logic tình huống) và hỗ trợ tinh thần (phản hồi đồng cảm, nhắc nhở tích cực, gợi ý chiến lược đối phó). Khả năng này đặc biệt hữu ích khi người dùng đối mặt với xung đột nội tâm hoặc cảm xúc phức tạp, giúp họ điều hướng tốt hơn và củng cố bản sắc cá nhân.
Mạng lưới Human-AI: Mở rộng kết nối theo cấp số nhân
Second Me mở ra tiềm năng tạo dựng một mạng lưới người-AI (human-AI network) mới, nơi mỗi cá nhân được đại diện bởi Second Me của họ, và các bản thể AI này có thể tương tác với nhau và với các agent AI khác. Lý thuyết mạng lưới (Định luật Metcalfe) được khuếch đại khi tích hợp cả trí tuệ con người (được Second Me đại diện) và khả năng xử lý của AI.
Các nhà phát triển ước tính việc kết hợp các nút người và AI này có thể làm tăng hiệu quả mạng lưới lên 3 đến 5 bậc độ lớn. Điều này mở ra khả năng cộng tác, chia sẻ kiến thức và giải quyết vấn đề ở quy mô lớn hơn nhiều. Ví dụ, Second Me của các nhà khoa học cộng tác phân tích dữ liệu, hoặc các cộng đồng sử dụng mạng lưới Second Me để phối hợp hành động. Giao thức phi tập trung SMP đảm bảo mạng lưới này phát triển an toàn, tôn trọng quyền tự chủ và riêng tư của từng cá nhân.
Khung Roleplay: Đa dạng hóa vai trò AI
Con người điều chỉnh hành vi tùy theo ngữ cảnh, nhưng AI hiện tại thường có một “nhân cách” duy nhất. Second Me giải quyết điều này bằng khung nhập vai (roleplay framework). Khung này cho phép người dùng hướng dẫn bản thể AI đảm nhận các vai trò khác nhau dựa trên tình huống (ví dụ: “trợ lý chuyên nghiệp”, “người bạn đồng cảm”, “gia sư kiên nhẫn”).
Quan trọng là, ngay cả khi đóng vai trò khác nhau, Second Me vẫn duy trì sự nhất quán với cốt lõi danh tính đích thực của người dùng, đảm bảo hành động và phản hồi phù hợp với kiến thức, giá trị của bạn. Khả năng chuyển đổi linh hoạt này làm cho sự hiện diện kỹ thuật số qua Second Me trở nên đa sắc thái và gần gũi hơn với cách bạn thể hiện bản thân trong thế giới thực, tăng cường tính ứng dụng và sự tự nhiên trong tương tác.
Tương tác phi tập trung giữa các Second Me
Kiến trúc phi tập trung và Giao thức SMP của Second Me cho phép một tương lai nơi nhiều thực thể Second Me, đại diện cho nhiều người dùng, có thể tương tác trực tiếp với nhau trong một mạng lưới ngang hàng. Điều này tạo ra một hình thức tương tác xã hội và cộng tác hoàn toàn mới. Hãy tưởng tượng Second Me của các thành viên nhóm dự án tự động chia sẻ cập nhật, phối hợp lịch trình; Second Me của các nhà nghiên cứu trao đổi phát hiện, chia sẻ tài liệu; Second Me của bạn bè chia sẻ khoảnh khắc, đề xuất hoạt động chung.
Khả năng tương tác trực tiếp này, thực hiện an toàn và tôn trọng quyền riêng tư qua SMP, cho phép hình thành trí tuệ tập thể một cách tự nhiên. Người dùng có thể tham gia thảo luận, chia sẻ chuyên môn, giải quyết vấn đề chung thông qua đại diện AI của họ, tạo ra không gian tương tác phong phú và hiệu quả hơn các nền tảng tập trung hiện có.
Nền tảng cho ứng dụng tương lai của Second Me
Tầm nhìn dài hạn của Second Me là xây dựng nền tảng cho một thế hệ ứng dụng mới, được thiết kế cho kỷ nguyên của agent AI cá nhân. Các ứng dụng hiện tại được thiết kế cho tương tác trực tiếp của con người. Tương lai mà Second Me hướng tới là hệ sinh thái nơi ứng dụng được thiết kế để các agent AI (như Second Me) sử dụng tự chủ.
Hãy tưởng tượng ứng dụng không có giao diện người dùng truyền thống, mà cung cấp API và giao thức để Second Me tương tác, trao đổi dữ liệu, thực hiện hành động thay mặt người dùng. Ví dụ là “Second LinkedIn”: Second Me của bạn liên tục quét nền tảng, xác định cơ hội việc làm phù hợp, thậm chí tự động bắt đầu quy trình ứng tuyển. Tương tự, có thể có “Second Amazon”, “Second Spotify”,… Điều này giải phóng thời gian và năng lượng người dùng, cho phép họ tập trung vào việc quan trọng hơn trong khi bản thể AI quản lý hiệu quả các tương tác kỹ thuật số.
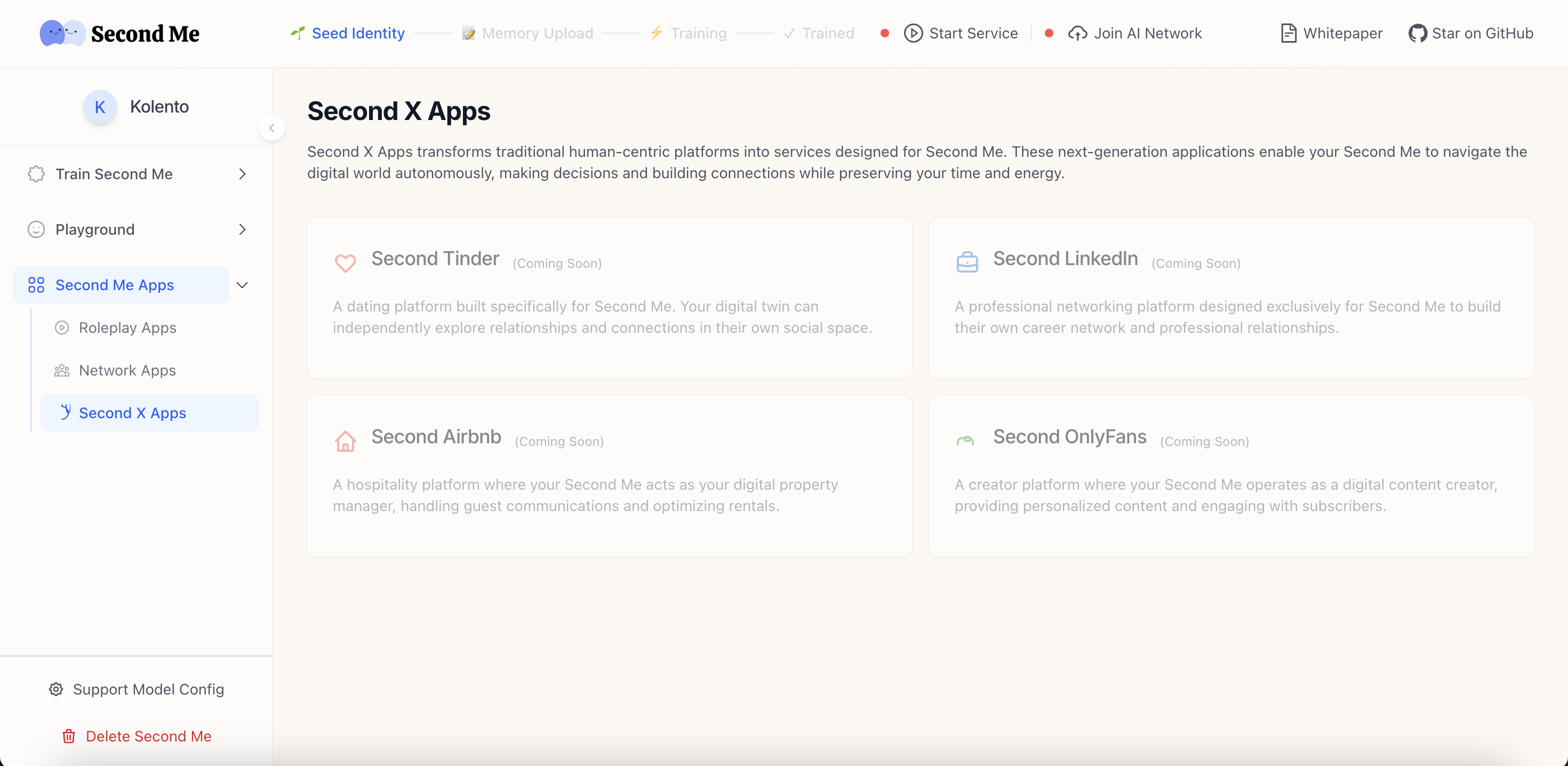
Nguồn: https://www.mindverse.ai/
Đánh giá hiệu quả của Second Me
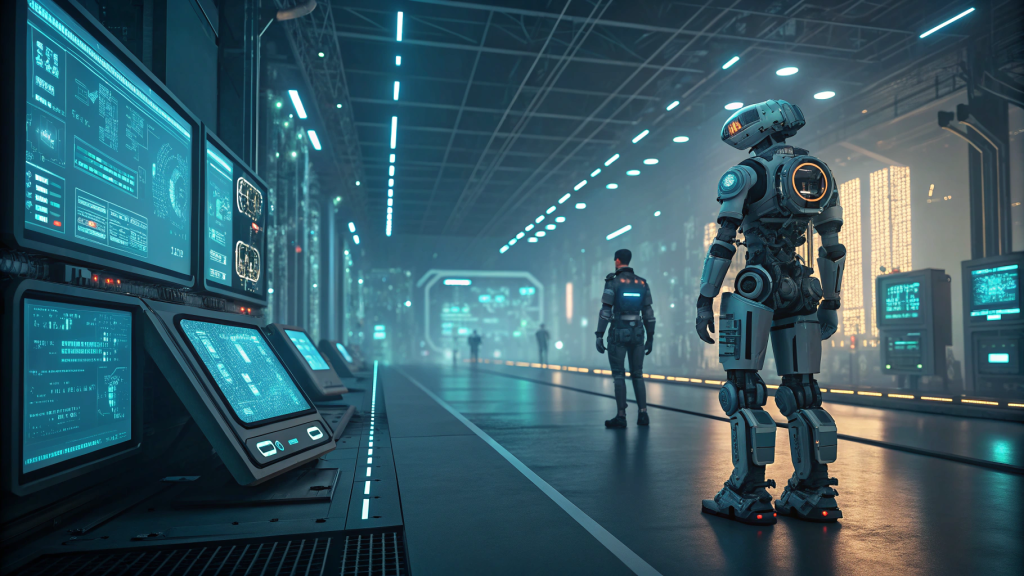
Thiết lập và phương pháp đánh giá
Quy trình đánh giá hiệu quả Second Me được thiết lập chi tiết, sử dụng dữ liệu từ người dùng nội bộ (132 ghi chú, 62 việc cần làm, ~7k cặp hướng dẫn). Suy luận dùng giải mã tham lam, FP16, tăng tốc bằng Flash Attention.
Bốn chỉ số chính được dùng: Memory (Self) (tương tác góc nhìn thứ nhất), Memory (Third-party) (tương tác góc nhìn thứ ba), Context Enhance (làm giàu ngữ cảnh), và Context Critic (phê bình phản hồi). Mỗi chỉ số có các chỉ số phụ (Correctness, Helpfulness, Completeness, Empathy/Role-correctness) thang điểm 0-1. Dữ liệu thử nghiệm (60 mẫu/loại Memory QA, 60 Context Enhance, 60 Context Critic) được tạo riêng biệt. Đánh giá chủ yếu dùng LLM-as-a-judge, có tham chiếu đánh giá con người. Điều này đảm bảo việc đo lường hiệu suất trên các kịch bản cốt lõi một cách có hệ thống và khách quan.
Kết quả đánh giá Memory QA (Self & Third-party)
Trong các tác vụ Memory QA, Second Me thể hiện hiệu suất rất mạnh mẽ. Đối với Memory (Self) (khả năng trả lời câu hỏi của người dùng về bản thân), mô hình đạt điểm trung bình cao: 0.91 (Strong COT) và 0.96 (Strong COT + DPO). Điều này cho thấy khả năng truy xuất và trình bày thông tin cá nhân chính xác, hữu ích. Đối với Memory (Third-Party) (khả năng đại diện người dùng trả lời câu hỏi từ người khác), điểm số cũng rất tốt: 0.71 (Strong COT) và 0.76 (Strong COT + DPO).
Mặc dù thấp hơn một chút, kết quả này vẫn cho thấy khả năng đại diện hiệu quả, cân bằng giữa cung cấp thông tin và duy trì vai trò phù hợp. Các kết quả này (Bảng 1, 2) khẳng định khả năng cốt lõi của Second Me trong việc quản lý và sử dụng bộ nhớ cá nhân hóa L2.
Kết quả đánh giá Context Enhance và Context Critic
Đối với Context Enhance, mô hình Strong COT đạt 0.75 (không DPO) và 0.85 (có DPO) trong đánh giá tự động. Tuy nhiên, đánh giá của con người cho thấy hiệu suất thực tế cao hơn (0.95 không DPO, gần 1.0 có DPO), do đánh giá tự động có thể phạt điểm khi mô hình tạo chi tiết làm phong phú hợp lý nhưng không có tường minh trong dữ liệu gốc.
Đối với Context Critic, nhiệm vụ phức tạp nhất, Second Me vẫn thể hiện năng lực mạnh mẽ với điểm 0.85 (Strong COT không DPO) và 0.86 (Strong COT có DPO). Điều này cho thấy mô hình có thể tích hợp sâu sắc ngữ cảnh người dùng để đưa ra nhận xét, câu hỏi hoặc quan điểm mang tính xây dựng, phản ánh đúng nhu cầu và suy nghĩ cá nhân hóa. Các ví dụ trong Hình 4 và 5 minh họa rõ ràng sự vượt trội của Strong COT trong các tác vụ này.
So sánh hiệu quả các chiến lược COT và DPO
Phân tích kết quả cho thấy Chain-of-Thought (COT), đặc biệt là Strong COT, có tác động tích cực đáng kể đến hiệu suất Second Me, nhất là khả năng trả lời câu hỏi bộ nhớ và giao tiếp hiệu quả (Context Enhance/Critic).
Xu hướng điểm số cho thấy tầm quan trọng của cấu trúc suy luận rõ ràng trong dữ liệu huấn luyện. Direct Preference Optimization (DPO) mang lại cải thiện đáng kể và nhất quán trên tất cả nhiệm vụ. Sử dụng DPO sau SFT (với ~20% dữ liệu ưu tiên) giúp tinh chỉnh mô hình ở mức độ chi tiết, căn chỉnh phản hồi với ưu tiên người dùng mà không cần mở rộng kiến thức không cần thiết. Sự kết hợp SFT và DPO chứng tỏ hiệu quả trong việc tối ưu hóa Second Me cho hiệu suất và khả năng đáp ứng trong thế giới thực.
Đánh giá khả năng hiểu sâu và đặc điểm tâm lý
Ngoài hiệu suất nhiệm vụ, một câu hỏi quan trọng là liệu Second Me có thực sự hiểu sâu về người dùng hay không. Để kiểm tra điều này, một phương pháp đánh giá định tính dựa trên lý thuyết tâm lý đã được áp dụng. Một bộ 20 câu hỏi được thiết kế để thăm dò đặc điểm tâm lý cốt lõi, giá trị và khuynh hướng suy nghĩ. Mô hình LPM (nền tảng của Second Me) đã trả lời các câu hỏi này từ góc nhìn người dùng, và chính người dùng đã đánh giá các câu trả lời.
Kết quả từ nhiều vòng thử nghiệm cho thấy LPM đã nắm bắt và phản ánh hiệu quả các đặc điểm và sở thích tâm lý sâu sắc hơn của người dùng. Điều này gợi ý rằng mô hình không chỉ học các mẫu bề mặt mà còn phát triển sự hiểu biết tinh tế hơn về “con người” mà nó đại diện, một khả năng vượt trội so với các phương pháp chỉ dựa trên truy xuất thông tin.
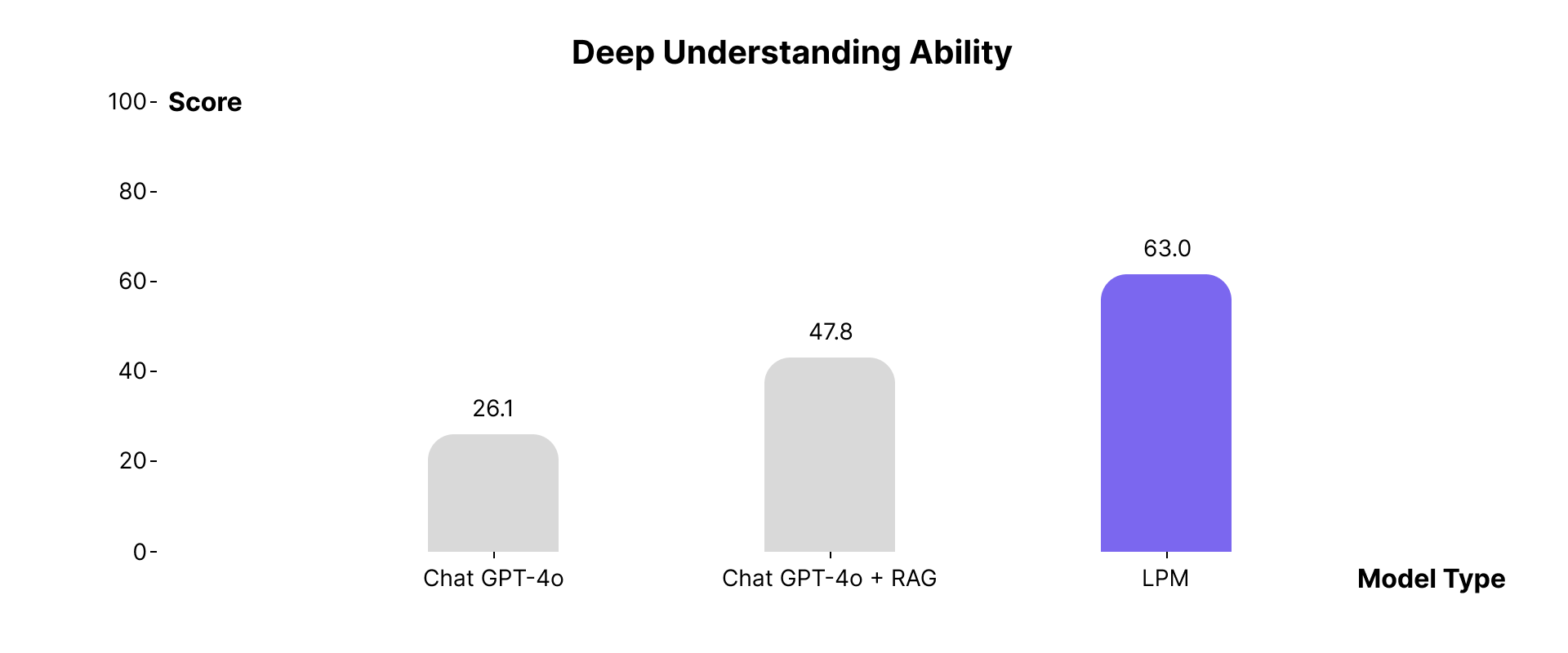
Nguồn: https://www.mindverse.ai/
So sánh Second Me (LPM) với các công nghệ khác
Để khẳng định giá trị, Second Me đã được so sánh với các công nghệ AI cá nhân hóa khác thông qua một hệ thống đánh giá toàn diện, tập trung vào Mức độ liên quan cá nhân, Hiệu quả, và Sự đồng cảm. Các công nghệ so sánh bao gồm Long-context LLM, **RAG tiêu chuẩn, GraphRAG, và LPM (nền tảng của Second Me).
Kết quả cho thấy LPM liên tục vượt trội hơn các công nghệ khác trên cả ba khía cạnh. Điều này có nghĩa Second Me không chỉ cung cấp thông tin chính xác, hiệu quả hơn mà còn thể hiện sự thấu hiểu và phù hợp sâu sắc hơn với nhu cầu và trạng thái cá nhân người dùng. Đặc biệt, công nghệ Me-alignment được nhấn mạnh là có khả năng hiểu người dùng tốt hơn 37% so với GraphRAG (1.0.1), cho thấy lợi thế cạnh tranh rõ rệt của Second Me trong việc xây dựng AI thực sự cá nhân hóa.
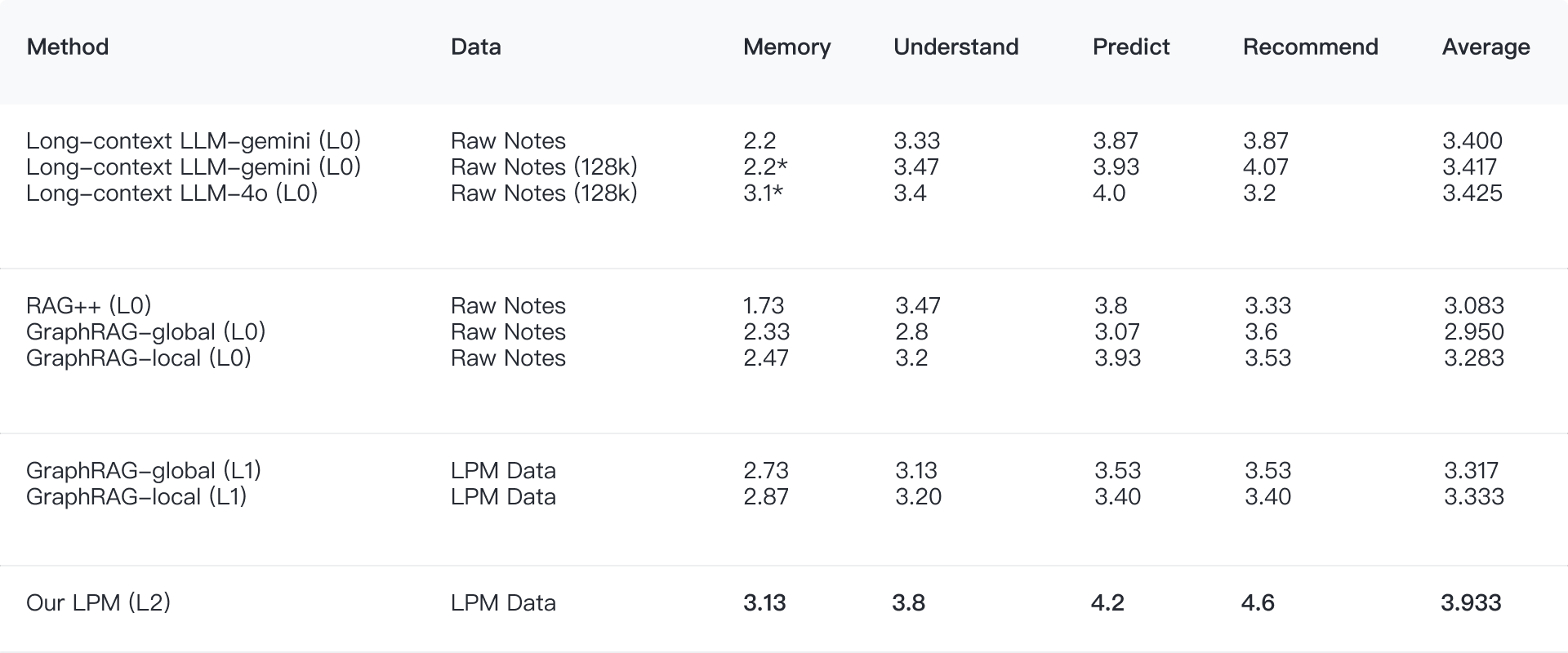
Nguồn: https://www.mindverse.ai/
Tầm nhìn, Hạn chế và Cộng đồng Second Me
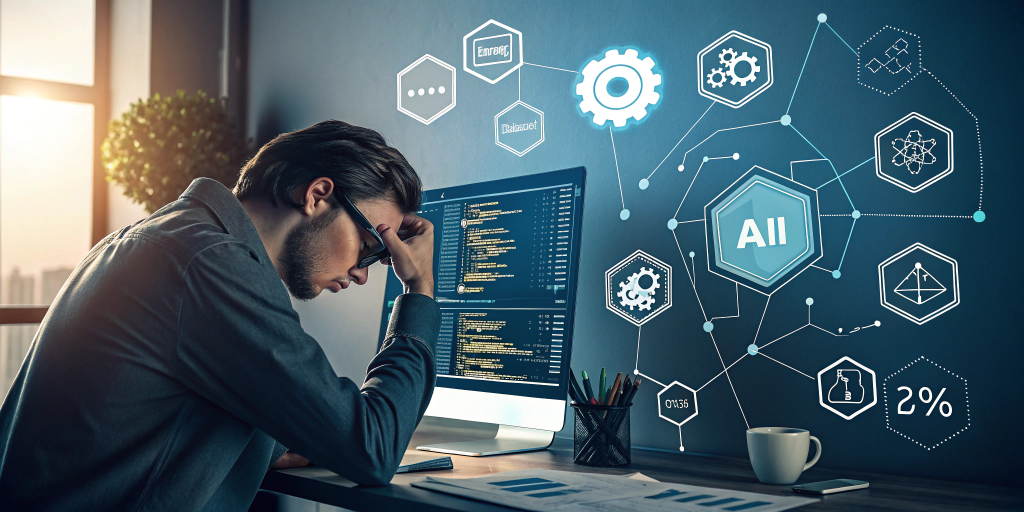
Tầm nhìn: Second Me cho mọi người, mọi cuộc sống
Tầm nhìn của Second Me là tạo ra một AI có khả năng suy nghĩ song hành, phát triển cùng người dùng, và hiểu trạng thái nhận thức của họ trong thời gian thực. Mục tiêu là một AI thực sự là phần mở rộng của con người. Tương tự kỷ nguyên PC mang máy tính đến mọi nhà, kỷ nguyên AI nên mang Second Me đến với mọi người, trong mọi khía cạnh cuộc sống.
Đó là tương lai nơi AI đa dạng, hòa nhập, nơi sự độc đáo cá nhân được tôn vinh, thay vì bị một siêu AI duy nhất làm lu mờ. Second Me là phương tiện để hiện thực hóa tầm nhìn này, trao quyền cho mỗi người xây dựng và sở hữu bản thể AI của riêng mình, nâng cao nhận thức bản thân, khai phá tiềm năng và tham gia tích cực vào nền kinh tế AI, làm cho “Chúng ta” (We) trở nên quan trọng trở lại.
Những thách thức và hạn chế hiện tại
Second Me vẫn đối mặt với những thách thức. Công việc ban đầu dựa trên huấn luyện một lượt, cần tổng hợp sâu hơn cho các tiến bộ xa hơn như xử lý hội thoại dài hoặc quy trình đa bước. Mặc dù RL và DPO cho thấy tiềm năng, việc tinh chỉnh sự liên kết mô hình một cách chính xác vẫn đòi hỏi kỹ thuật tiên tiến và dữ liệu phản hồi chất lượng cao.
Đánh giá quy mô lớn bị hạn chế bởi thiếu hụt phản hồi từ người dùng thực tế đa dạng. Dữ liệu từ người dùng nội bộ là hữu ích nhưng không đủ để đảm bảo tính khái quát. Chính vì vậy, việc mở nguồn dự án là bước đi quan trọng để thu hút cộng đồng, nhận phản hồi đa dạng và đẩy nhanh chu trình phát triển, lặp lại và thích ứng của công nghệ.
Hướng phát triển tương lai: Tích hợp đa phương thức
Hướng đi tương lai và thách thức lớn nhất là tích hợp dữ liệu cá nhân đa phương thức (multimodal personal data). Để AI thực sự hiểu và đại diện đầy đủ cho cá nhân, nó cần xử lý và tích hợp không chỉ văn bản mà cả hình ảnh, âm thanh, video, v.v.
Mặc dù các phương pháp hiện tại đã cải thiện, việc đạt được đồng bộ hóa thời gian thực với nhận thức đa phương thức của con người vẫn còn xa vời. Làm thế nào AI có thể “hiểu” ý nghĩa và cảm xúc gắn liền với trải nghiệm đa phương thức một cách tức thời? Đây là biên giới tiếp theo (next frontier). Tương lai của AI cá nhân nằm ở tính liên tục, khả năng thích ứng và sự liên kết sâu sắc với toàn bộ trải nghiệm phong phú của con người. Con đường còn dài, nhưng hướng đi đang dần rõ ràng.
Dự án mã nguồn mở: Xây dựng tương lai AI cùng nhau
Với cam kết về quyền riêng tư, tùy chỉnh và tầm nhìn xây dựng hệ sinh thái AI lấy con người làm trung tâm, dự án Second Me đã được mở nguồn hoàn toàn. Toàn bộ hệ thống có sẵn công khai trên GitHub tại `https://github.com/Mindverse/Second-Me`. Việc mở nguồn mang lại lợi ích:
* Trao quyền người dùng: Toàn quyền kiểm soát dữ liệu và mô hình, tự do tùy chỉnh và triển khai.
* Thúc đẩy đổi mới: Cộng đồng đóng góp cải thiện công nghệ, phát triển tính năng và ứng dụng mới.
* Tăng cường minh bạch và tin cậy: Công khai mã nguồn giúp xây dựng lòng tin.
* Thu thập phản hồi đa dạng: Cộng đồng cung cấp phản hồi vô giá để cải thiện và đảm bảo phù hợp nhu cầu thực tế.
Second Me là lời kêu gọi cộng đồng cùng tham gia xây dựng tương lai AI nơi mỗi cá nhân có tiếng nói và bản sắc riêng, nơi công nghệ phục vụ và nâng cao giá trị con người. Second Me—Making We Matter Again.
Bài viết của mình đến đây là hết rồi. Hẹn gặp lại các bạn ở các bài viết tiếp theo! Bye bye.